Common Data Analysis Pitfalls to Avoid
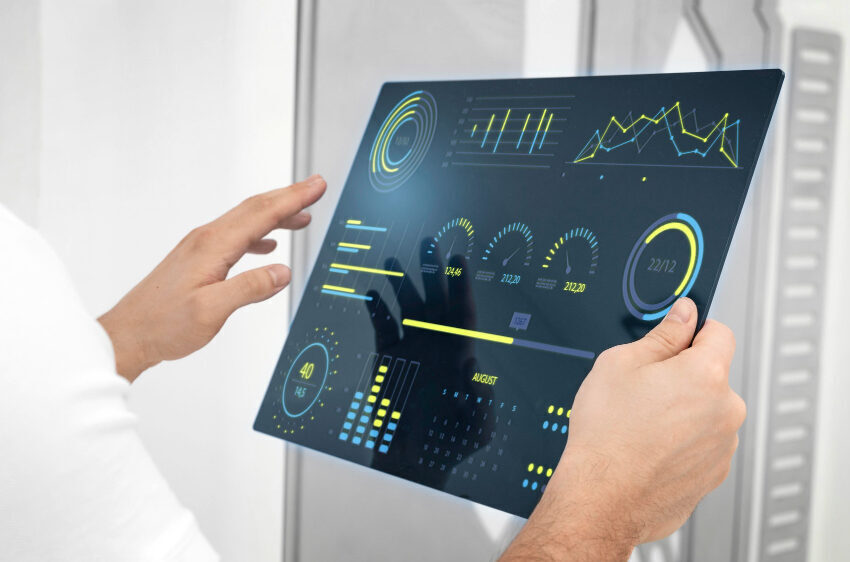
Data analysis is an essential part of decision-making in any business, but without careful attention, it’s easy to fall into common pitfalls that can lead to inaccurate conclusions, wasted resources, or missed opportunities. In this blog, we will explore some of the most common mistakes organizations make when conducting data analysis and how to avoid them to ensure you’re deriving meaningful and actionable insights from your data.
1. Ignoring Data Quality
One of the most critical factors in successful data analysis is the quality of the data. Poor-quality data, whether it’s incomplete, outdated, or inaccurate, can lead to misleading insights and incorrect decisions. Many organizations make the mistake of jumping into analysis without addressing data quality, leading to results that may not reflect the true situation.
How to Avoid It:
Before conducting any analysis, take the time to clean and validate your data. Implement data quality checks to ensure completeness, accuracy, and consistency. Regularly review and update data sources to avoid issues caused by outdated or incorrect information.
2. Not Defining Clear Objectives
Data analysis should always have a clear objective or question in mind. Without a defined goal, it’s easy to get lost in the data and perform analysis without any meaningful direction. A lack of clarity on what you’re trying to achieve can result in analyzing irrelevant data, wasting time, and deriving insights that don’t support your business objectives.
How to Avoid It:
Before diving into analysis, define a clear research question or business objective. What specific problem are you trying to solve or what decision are you trying to inform? This helps to focus your efforts and ensures that the insights you gain will be relevant and actionable
3. Overlooking Data Bias
Bias is one of the most subtle and dangerous issues in data analysis. Data can be biased in various ways, such as from sampling errors, measurement biases, or even the way data is collected and interpreted. If bias is not recognized and accounted for, the analysis may not represent the reality, leading to flawed conclusions.
How to Avoid It:
Examine the data for potential sources of bias. Make sure your sample is representative and that the data collection methods are objective and consistent. Be aware of any biases that may affect how data is interpreted or presented and strive to eliminate or adjust for them in your analysis.
4. Focusing Too Much on Correlation Instead of Causation
One of the most common mistakes in data analysis is assuming that because two variables are correlated, one must cause the other. Correlation does not imply causation, and overlooking this distinction can lead to false conclusions and poor decisions.
How to Avoid It:
While correlation analysis is valuable, ensure you are not jumping to conclusions about causality. Use statistical techniques, such as regression analysis or experimental design, to explore and understand the underlying relationships between variables more accurately.
5. Overcomplicating the Analysis
In some cases, analysts can become so focused on using complex techniques and models that they lose sight of the core objective. Overcomplicating the analysis can not only confuse stakeholders but also lead to overfitting, where the model works perfectly on the training data but fails to generalize to new data.
How to Avoid It:
Stick to the simplest model that provides useful insights. Focus on the key variables and metrics that directly address your business question. If a more complex model is necessary, ensure that it adds clear value and that the additional complexity justifies the benefits.
6. Neglecting to Visualize Data
Data analysis often involves working with large datasets, and it’s easy to get caught up in numbers and statistics. However, without proper visualization, it can be challenging to communicate insights effectively to non-technical stakeholders. Failing to visualize data can make it harder for others to understand the analysis and act on it.
How to Avoid It:
Invest time in creating clear and meaningful visualizations. Use graphs, charts, and dashboards that highlight key insights and trends in the data. Well-designed visuals make it easier to spot patterns, trends, and outliers, enabling better decision-making and understanding across the organization.
7. Relying Too Heavily on Historical Data
While historical data can provide valuable insights, relying too heavily on it can be problematic. Business environments and market conditions can change rapidly, and past trends may not always be indicative of future performance. Over-relying on historical data can lead to outdated conclusions and missed opportunities.
How to Avoid It:
Incorporate predictive analytics and real-time data into your analysis. While historical data provides a foundation, augment it with forecasting models and current data to gain a more accurate picture of future trends and dynamics.
8. Failure to Communicate Findings Effectively
Even the most accurate and insightful data analysis is of little value if it’s not communicated properly. Analysts sometimes fail to effectively translate their findings into clear, actionable insights for decision-makers, resulting in confusion or inaction.
How to Avoid It:
Focus on clear communication of your findings. Tailor your reports and presentations to your audience, highlighting key insights and their implications for the business. Use simple language and visualizations to convey your points effectively and ensure your audience can take appropriate action.
9. Ignoring the Importance of Context
Data doesn’t exist in a vacuum, and without proper context, it’s easy to misinterpret results. Analyzing data without understanding the broader context—such as market conditions, industry trends, or organizational goals—can lead to incomplete or misguided conclusions.
How to Avoid It:
Always consider the context in which the data was collected and analyzed. Understand the external factors that could influence the results, and use this context to provide a fuller picture when interpreting the data.
10. Not Iterating or Updating the Analysis
Data analysis isn’t a one-time task. As new data comes in or as business needs evolve, it’s essential to revisit and update analyses regularly. Failing to do so can lead to decisions based on outdated information or assumptions that no longer hold true.
How to Avoid It:
Make data analysis an ongoing process. Regularly update your models, re-analyze data, and refine your insights as new information becomes available. This ensures that your decisions remain based on the most current and relevant data.
Conclusion
Avoiding common data analysis pitfalls is essential for extracting actionable, valuable insights from your data. By ensuring data quality, defining clear objectives, recognizing bias, and using the right visualization tools, organizations can make more informed decisions, optimize processes, and improve business outcomes. Continuous learning and iteration are key to improving your analysis and staying ahead in today’s data-driven world.